|
ACCESS THE FULL ARTICLE
No SPIE Account? Create one
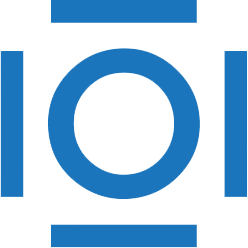
CITATIONS
Cited by 3 scholarly publications.
Sensors
Roads
Global Positioning System
Cameras
Data acquisition
Stereoscopic cameras
Evolutionary algorithms