|
ACCESS THE FULL ARTICLE
No SPIE Account? Create one
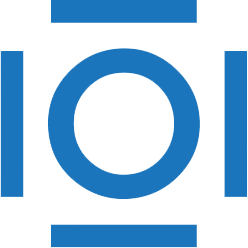
CITATIONS
Cited by 27 scholarly publications.
Sensors
Target detection
Detection and tracking algorithms
Hyperspectral imaging
Contamination
Scanning electron microscopy
Expectation maximization algorithms