|
ACCESS THE FULL ARTICLE
No SPIE Account? Create one
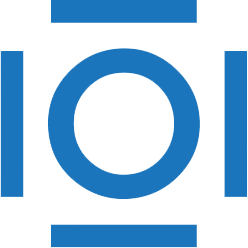
CITATIONS
Cited by 4 scholarly publications.
Associative arrays
Chemical species
Denoising
Image denoising
Algorithm development
Cameras
Matrices