|
ACCESS THE FULL ARTICLE
No SPIE Account? Create one
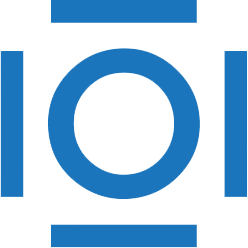
CITATIONS
Cited by 3 scholarly publications and 1 patent.
Lawrencium
Super resolution
Neural networks
Image restoration
Imaging systems
Image filtering
Image processing