In recent years, skeleton-based action recognition has become increasingly popular in the field of human action recognition, and graph convolutional networks (GCNs) have shown better advantages in this task. Many GCN-based methods are insufficient in the latent relationship between features, which affects the discriminability of features being not rich enough. These potential feature relationships can manifest as feature differences that change due to actions and feature correlations that interact with each other. Therefore, we propose a feature difference and feature correlation learning mechanism to learn discriminative augmentation features, including feature differences in actions and feature correlations between joints. First, we propose a temporal feature difference and correlation learning module (FDCL) (TFDCL). In adjacent temporal frames, we extract feature correlations between related parts. Feature differences are captured through changes in joints over the overall long-term timeline. Second, we propose a channel FDCL module. Different channels contain different types of features for actions. We use convolution operations to interact between channels, continuously extracting the strongest features to obtain feature maps. Third, we propose a temporal channel context topology (TCCT) module to dynamically learn global contextual features of all joints during motion. Finally, experiments are conducted on the NTU-RGBD 60 dataset and the kinetics-skeleton 400 dataset to verify the effectiveness of the network. |
ACCESS THE FULL ARTICLE
No SPIE Account? Create one
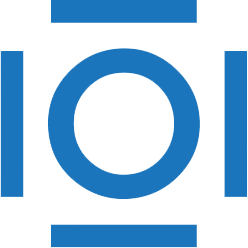
CITATIONS
Cited by 1 scholarly publication.
Action recognition
Feature extraction
Convolution
Education and training
Bone
Matrices
Data modeling