|
ACCESS THE FULL ARTICLE
No SPIE Account? Create one
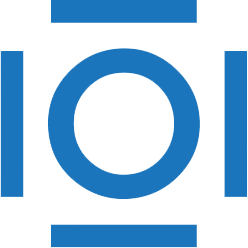
CITATIONS
Cited by 6 scholarly publications.
Frequency modulation
Fermium
Signal to noise ratio
Radar
Signal detection
Neural networks
Interference (communication)