|
ACCESS THE FULL ARTICLE
No SPIE Account? Create one
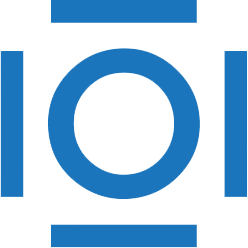
CITATIONS
Cited by 1 scholarly publication and 1 patent.
Neural networks
Cameras
Network architectures
Neurons
Embedded systems
Image resolution
Matrices