|
ACCESS THE FULL ARTICLE
No SPIE Account? Create one
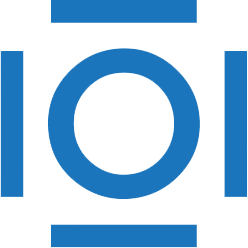
CITATIONS
Cited by 1 scholarly publication.
Data modeling
Image fusion
Inverse problems
Optimization (mathematics)
Algorithm development
Convex optimization
Deconvolution