|
ACCESS THE FULL ARTICLE
No SPIE Account? Create one
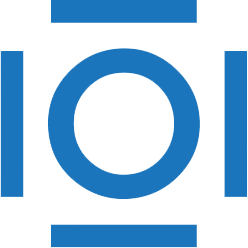
CITATIONS
Cited by 1 scholarly publication.
Data modeling
Tissues
Head
Magnetic resonance imaging
Tumor growth modeling
Motion models
Scanners