|
ACCESS THE FULL ARTICLE
No SPIE Account? Create one
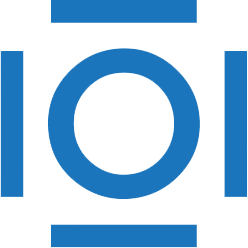
CITATIONS
Cited by 5 scholarly publications.
Image segmentation
Roads
Sensors
Computer programming
Network architectures
Convolution
Image classification