|
ACCESS THE FULL ARTICLE
No SPIE Account? Create one
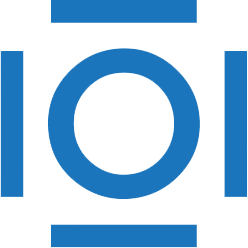
CITATIONS
Cited by 1 scholarly publication.
Databases
Content addressable memory
Binary data
Neurons
Performance modeling
Data modeling
Data storage