|
ACCESS THE FULL ARTICLE
No SPIE Account? Create one
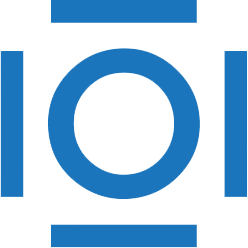
CITATIONS
Cited by 2 scholarly publications.
Reliability
Analytical research
Prototyping
Data processing
Mathematical modeling
Data fusion
Sensors