|
ACCESS THE FULL ARTICLE
No SPIE Account? Create one
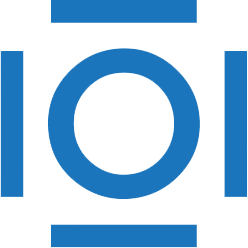
CITATIONS
Cited by 1 scholarly publication.
Image segmentation
Liver
Tumors
Performance modeling
Medical imaging
Data modeling
Image filtering