|
ACCESS THE FULL ARTICLE
No SPIE Account? Create one
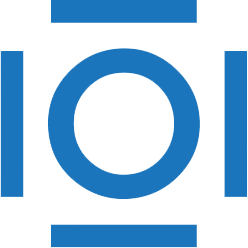
CITATIONS
Cited by 2 scholarly publications.
Optimization (mathematics)
Binary data
Distance measurement
Statistical modeling
Manufacturing