|
ACCESS THE FULL ARTICLE
No SPIE Account? Create one
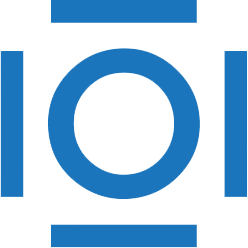
CITATIONS
Cited by 5 scholarly publications.
Computed tomography
Convolutional neural networks
Feature extraction
Performance modeling
Convolution
Visual process modeling
X-ray computed tomography