|
ACCESS THE FULL ARTICLE
No SPIE Account? Create one
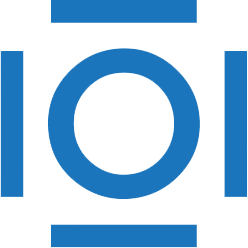
CITATIONS
Cited by 1 scholarly publication.
Image classification
Convolutional neural networks
RGB color model
Neurons
Optimization (mathematics)
Tumors
Bladder cancer