|
ACCESS THE FULL ARTICLE
No SPIE Account? Create one
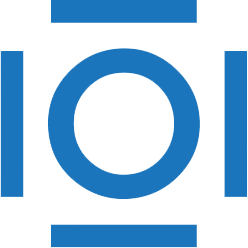
CITATIONS
Cited by 5 scholarly publications.
Image segmentation
Computer programming
Image processing algorithms and systems
Image visualization
Network architectures
Data modeling
Visualization