|
ACCESS THE FULL ARTICLE
No SPIE Account? Create one
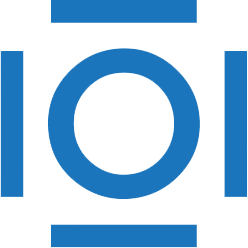
CITATIONS
Cited by 1 scholarly publication.
Vegetation
Convolutional neural networks
Image classification
Remote sensing
Satellites
Calibration
Multispectral imaging