|
ACCESS THE FULL ARTICLE
No SPIE Account? Create one
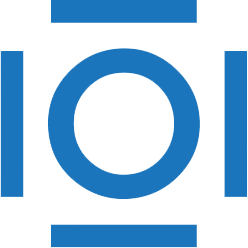
CITATIONS
Cited by 1 scholarly publication.
Machine learning
Robots
RGB color model
Cameras
Detection and tracking algorithms
Unmanned ground vehicles
Convolution