|
ACCESS THE FULL ARTICLE
No SPIE Account? Create one
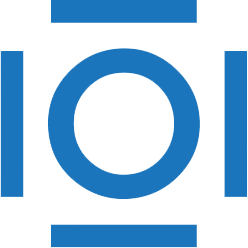
CITATIONS
Cited by 14 scholarly publications.
Network architectures
Head
Neurons
Convolution
Facial recognition systems
Reliability