|
ACCESS THE FULL ARTICLE
No SPIE Account? Create one
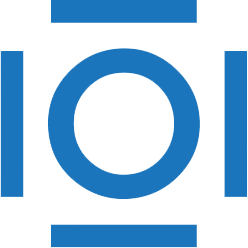
CITATIONS
Cited by 1 scholarly publication.
Neural networks
Error analysis
Data modeling
Network architectures
Artificial neural networks
Aluminum
Molybdenum