|
ACCESS THE FULL ARTICLE
No SPIE Account? Create one
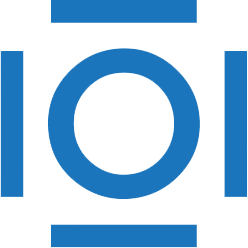
CITATIONS
Cited by 2 scholarly publications.
Control systems
Fuzzy logic
Device simulation
Genetic algorithms
Mathematical modeling
Systems modeling
Artificial neural networks