|
ACCESS THE FULL ARTICLE
No SPIE Account? Create one
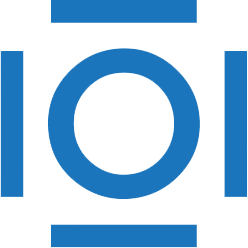
CITATIONS
Cited by 2 scholarly publications.
Diagnostics
Neurons
Algorithm development
Rule based systems
Statistical analysis
Neural networks
Classification systems