|
ACCESS THE FULL ARTICLE
No SPIE Account? Create one
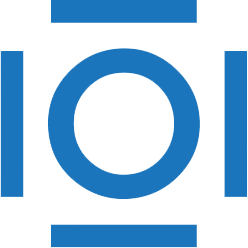
CITATIONS
Cited by 4 scholarly publications.
Optical correlators
Neural networks
Detection and tracking algorithms
Image filtering
Error analysis
Databases
Synthetic aperture radar