|
ACCESS THE FULL ARTICLE
No SPIE Account? Create one
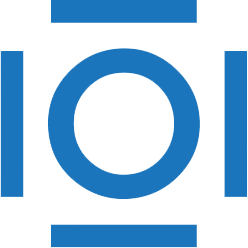
CITATIONS
Cited by 3 scholarly publications.
Neurons
Synthetic aperture radar
Neural networks
Automatic target recognition
Target detection
Algorithm development
Data modeling