|
ACCESS THE FULL ARTICLE
No SPIE Account? Create one
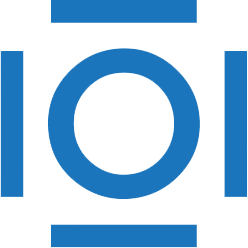
CITATIONS
Cited by 7 scholarly publications.
Hyperspectral imaging
Sensors
Reconstruction algorithms
Principal component analysis
Transform theory
Data compression
Data modeling