|
ACCESS THE FULL ARTICLE
No SPIE Account? Create one
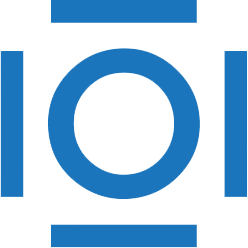
CITATIONS
Cited by 1 scholarly publication.
Detection and tracking algorithms
Wavelets
Dimension reduction
Neural networks
Hyperspectral imaging
Principal component analysis
Algorithms