|
ACCESS THE FULL ARTICLE
No SPIE Account? Create one
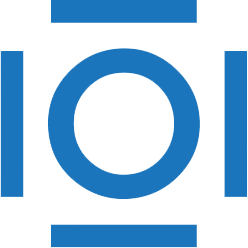
CITATIONS
Cited by 2 scholarly publications.
Statistical analysis
Radiometry
Data modeling
Roads
Sensors
Vegetation
Remote sensing