|
ACCESS THE FULL ARTICLE
No SPIE Account? Create one
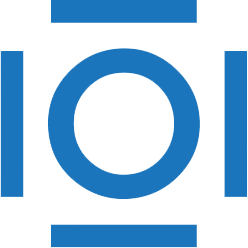
CITATIONS
Cited by 3 patents.
Data modeling
Remote sensing
Earth observing sensors
Landsat
Spherical lenses
Ecosystems
Agriculture