|
ACCESS THE FULL ARTICLE
No SPIE Account? Create one
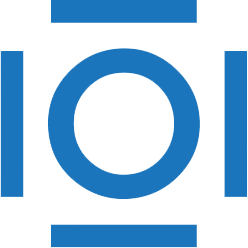
CITATIONS
Cited by 2 scholarly publications.
Neural networks
Superposition
Deconvolution
Network architectures
Neurons
Visual process modeling
Evolutionary algorithms