|
ACCESS THE FULL ARTICLE
No SPIE Account? Create one
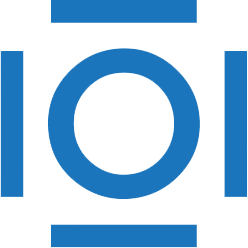
CITATIONS
Cited by 43 scholarly publications and 2 patents.
Expectation maximization algorithms
Reconstruction algorithms
Evolutionary algorithms
Detection and tracking algorithms
Image restoration
Sensors
Denoising