|
ACCESS THE FULL ARTICLE
No SPIE Account? Create one
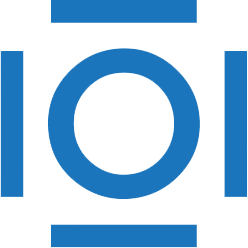
CITATIONS
Cited by 2 scholarly publications.
Gases
Long wavelength infrared
Sensors
Data modeling
Absorption
Hyperspectral imaging
Atmospheric sensing