|
ACCESS THE FULL ARTICLE
No SPIE Account? Create one
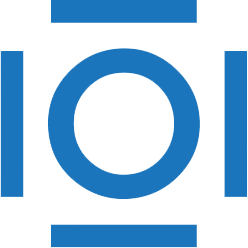
CITATIONS
Cited by 2 scholarly publications.
Raman spectroscopy
Artificial neural networks
Neurons
Molecules
Complex systems
Signal processing
Biological research