|
ACCESS THE FULL ARTICLE
No SPIE Account? Create one
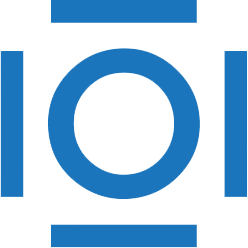
CITATIONS
Cited by 1 scholarly publication.
Wavelets
Neural networks
Neurons
Discrete wavelet transforms
Wavelet transforms
Evolutionary algorithms
Linear filtering