|
ACCESS THE FULL ARTICLE
No SPIE Account? Create one
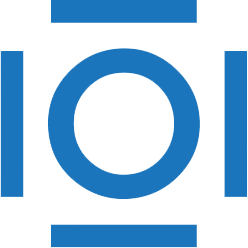
CITATIONS
Cited by 1 scholarly publication.
Biomedical optics
Associative arrays
Feature extraction
Binary data
Error analysis
Medicine
Pattern recognition