|
ACCESS THE FULL ARTICLE
No SPIE Account? Create one
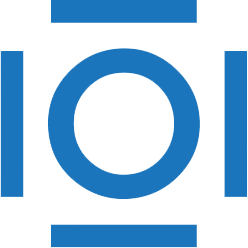
CITATIONS
Cited by 3 scholarly publications.
Hyperspectral imaging
Associative arrays
Surface plasmons
Data modeling
Signal to noise ratio
Chemical elements
Convex optimization