|
ACCESS THE FULL ARTICLE
No SPIE Account? Create one
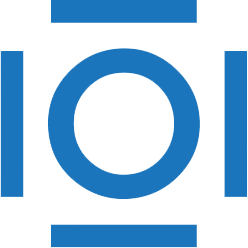
CITATIONS
Cited by 4 scholarly publications.
Magnetic resonance imaging
Image segmentation
Brain
3D modeling
Tumors
Tissues
Expectation maximization algorithms