|
ACCESS THE FULL ARTICLE
No SPIE Account? Create one
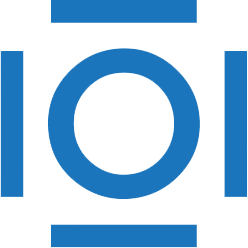
CITATIONS
Cited by 2 scholarly publications.
Principal component analysis
Data modeling
Machine learning
Feature selection
Mathematical modeling
Statistical modeling
Databases