|
ACCESS THE FULL ARTICLE
No SPIE Account? Create one
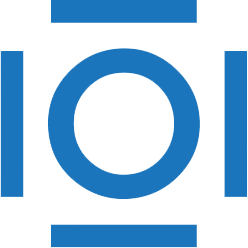
CITATIONS
Cited by 2 scholarly publications.
Composites
Hyperspectral imaging
Associative arrays
Algorithm development
Data modeling
Spatial resolution
Spectral resolution