|
ACCESS THE FULL ARTICLE
No SPIE Account? Create one
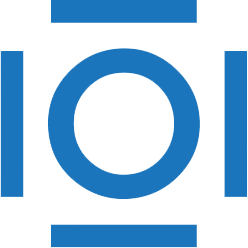
CITATIONS
Cited by 3 scholarly publications.
Diffusion
Visual process modeling
RGB color model
Visualization
Data modeling
Image segmentation
Computer vision technology