|
ACCESS THE FULL ARTICLE
No SPIE Account? Create one
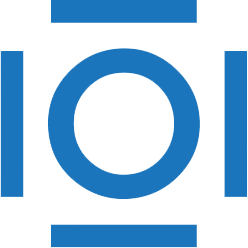
CITATIONS
Cited by 4 scholarly publications.
Feature selection
Image segmentation
Feature extraction
Mammography
Breast cancer
Image classification
Computer aided diagnosis and therapy