|
ACCESS THE FULL ARTICLE
No SPIE Account? Create one
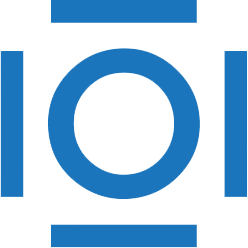
CITATIONS
Cited by 1 scholarly publication.
Detection and tracking algorithms
Target detection
Associative arrays
Hyperspectral imaging
Hyperspectral target detection
Performance modeling
Data modeling