|
ACCESS THE FULL ARTICLE
No SPIE Account? Create one
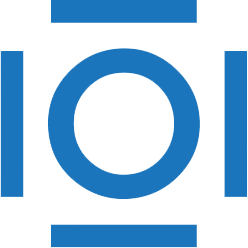
CITATIONS
Cited by 5 scholarly publications.
Roads
Unattended ground sensors
Unmanned aerial vehicles
Data modeling
Data fusion
Motion models
Sensors