|
ACCESS THE FULL ARTICLE
No SPIE Account? Create one
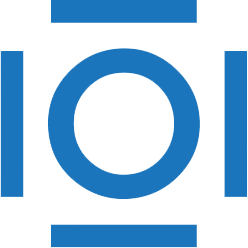
CITATIONS
Cited by 2 scholarly publications.
Transform theory
Magnetic resonance imaging
Compressed sensing
Reconstruction algorithms
Associative arrays
Image restoration
Data acquisition