|
ACCESS THE FULL ARTICLE
No SPIE Account? Create one
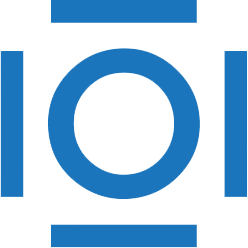
CITATIONS
Cited by 5 scholarly publications.
Autoregressive models
Composites
Sensors
Stochastic processes
Sensor networks
Data modeling
Unmanned aerial vehicles