|
ACCESS THE FULL ARTICLE
No SPIE Account? Create one
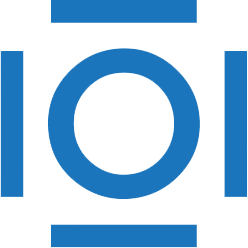
CITATIONS
Cited by 12 scholarly publications.
Data modeling
Snow cover
Raman spectroscopy
Earth observing sensors
Machine learning
Landsat
Performance modeling