|
ACCESS THE FULL ARTICLE
No SPIE Account? Create one
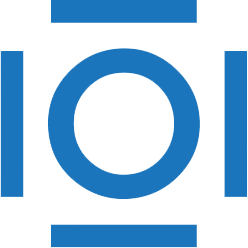
CITATIONS
Cited by 9 scholarly publications.
Remote sensing
Principal component analysis
Statistical modeling
Binary data
Spatial resolution
Feature extraction
Denoising