Forest has complex horizontal and vertical structures, so it is difficult to accurately identify tree species from remote sensing images only by spectral characteristics. Based on the airborne hyperspectral and LiDAR data sets, adding spatial features into tree species identifying procedure was studied in Gaofeng Forest Farm of Guangxi, China. As a result, a tree species classification method of synergizing spectral, spatial, and vertical structure features was proposed. First, principal components (PCs) were extracted from the hyperspectral data acquired by the AISA Eagle II sensor as spectral features. Four typical spatial features, gray level co-occurrence matrix, extended morphological profile, extended multiattribute profile, and multiscale guided filtering (MGF), were derived from the principal component bands. Meanwhile, digital elevation model, digital surface model (DSM), and normalized DSM were calculated from the LiDAR data to represent the characteristics of vertical structure. Then 13 spectral–spatial–vertical feature combinations were built and compared to classify the tree species using random forest classifier. The experimental results show that both spatial and vertical structure features can effectively improve the classification accuracy of tree species when banding with spectral features, and the feature combinations including multiscale spatial features is more conducive to improve classification accuracy. Compared with the method of using spectral features only, the methods with spatial features or vertical structure features could enhance the overall accuracy (OA) maximally by 49.49% and 14.65%. Among all the feature combinations, the synergy of PC bands, DSM, and MGF features achieves the highest classification accuracy, with OA 96.10% and kappa 0.9575. The spatial features have a great application potential for tree species classification in a plantation forest environment. |
ACCESS THE FULL ARTICLE
No SPIE Account? Create one
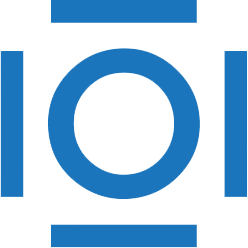
CITATIONS
Cited by 10 scholarly publications.
LIDAR
Feature extraction
Electroactive polymers
Image classification
Hyperspectral imaging
Data modeling
Reflectivity