Recently, many convolutional neural networks (CNN) have been successfully employed in bitemporal synthetic aperture radar (SAR) image change detection. However, most of the existing networks are too heavy and occupy a large volume of memory for storage and calculation. Motivated by this, we propose a lightweight neural network to reduce the computational and spatial complexity and facilitate the change detection on an edge device. We replace normal convolutional layers with bottleneck layers that keep the same number of channels between input and output. Next, we employ dilated convolutional kernels with a few non-zero entries that reduce the running time in convolutional operators. Comparing with the conventional convolutional neural network, our lightweight neural network will be more efficient with fewer parameters. We verify our light-weighted neural network on four sets of bitemporal SAR images. The experimental results show that the proposed network can obtain better performance than the conventional CNN and has better model generalization, especially on the challenging datasets with complex scenes. |
ACCESS THE FULL ARTICLE
No SPIE Account? Create one
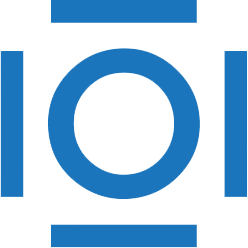
CITATIONS
Cited by 9 scholarly publications.
Synthetic aperture radar
Convolutional neural networks
Convolution
Neural networks
Computer programming
Visualization
Speckle