Semantic segmentation is an important and foundational task in the application of high-resolution remote sensing images (HRRSIs). However, HRRSIs feature large differences within categories and minor variances across categories, posing a significant challenge to the high-accuracy semantic segmentation of HRRSIs. To address this issue and obtain powerful feature expressiveness, a deep conditional generative adversarial network (DCGAN), integrating fully convolutional DenseNet (FC-DenseNet) and Pix2pix, is proposed. The DCGAN is composed of a generator–discriminator pair, which is built on a modified downsampling unit of FC-DenseNet. The proposed method possesses strong feature expression ability because of its skip connections, the very deep network structure and multiscale supervision introduced by FC-DenseNet, and the supervision from the discriminator. Experiments on a Deep Globe Land Cover dataset demonstrate the feasibility and effectiveness of this approach for the semantic segmentation of HRRSIs. The results also reveal that our method can mitigate the influence of class imbalance. Our approach for precise semantic segmentation can effectively facilitate the application of HRRSIs. |
ACCESS THE FULL ARTICLE
No SPIE Account? Create one
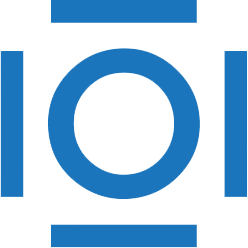
CITATIONS
Cited by 6 scholarly publications and 1 patent.
Image segmentation
Convolution
Data modeling
Remote sensing
Agriculture
Network architectures
Satellites